Signal/Data Processing & Analysis
The problems of signal and image processing are ubiquitous in the engineering, science, and healthcare fields. This is a genre of problem at the core of Barron Associates’ capabilities since its founding in 1984. As a pioneer in neural networks and, in particular, neural network-based adaptive control, Barron Associates has a vast library of tools and decades of experience in the challenging problems of signal and image processing.
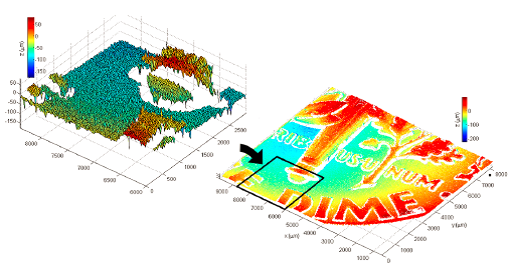
Figure 1: Surface Feature Extracted from Noisy Measurement Data
Barron Associates’ core competencies are reflected in its GNOSIS and APIMS software tools; however, Barron Associates has many more in-house software libraries for application to challenging customer problems. A partial list of Barron Associates competencies and techniques includes:
- Physics-based dynamic system model development
- Neural Networks for estimation and classification
- Stochastic modeling and uncertainty quantification/representation
- Unscented Kalman filters and particle filters
- Maximum likelihood estimation
- Nonlinear system identification
Typical applications include:
- Pattern recognition and classification
- Automatic target identification and tracking
- Statistical change detection
- Data mining
- Fault diagnosis and condition based maintenance
- Data smoothing and reconstruction
Related Project
A Tool to Predict Hot Corrosion of Nickel-based Turbine Disks
Hot Corrosion of turbine engine components has been studied for many years. The underlying mechanisms of Type II Hot Corrosion (Low-Temperature Hot Corrosion) are increasingly well-understood.... View ProjectResources
- M. DeVore and A. Bateman, “Polynomial chaos theory for performance evaluation of ATR systems,” Automatic Target Recognition XX, Proc. of SPIE, vol. 7696, (Orlando, FL), May 2010.
- X. Zhou and M. D. DeVore, “Data and model accuracy requirements analysis for target recognition from ladar data,” IEEE Trans. on Aerospace and Electronic Systems, vol. 44, pp. 1416-1432, 2008.
- M.D. DeVore, “Estimates of Error Probability for Complex Gaussian Channels with Generalized Likelihood Ratio Detection,” IEEE Trans. Pattern Analysis and Machine Intelligence, vol. 27, pp. 1580–1591, Oct. 2005.
- M.D. DeVore and J.A. O’Sullivan, “Quantitative Statistical Assessment of Conditional Models for Synthetic Aperture Radar,” IEEE Trans. on Image Processing, vol. 13, pp. 113–125, Feb. 2004.